By Andrea Pellandra, Senior Data Scientist, and Geraldine Henningsen, Data Scientist, UNHCR
➡️ Read the published article on the study in the International Migration Review.
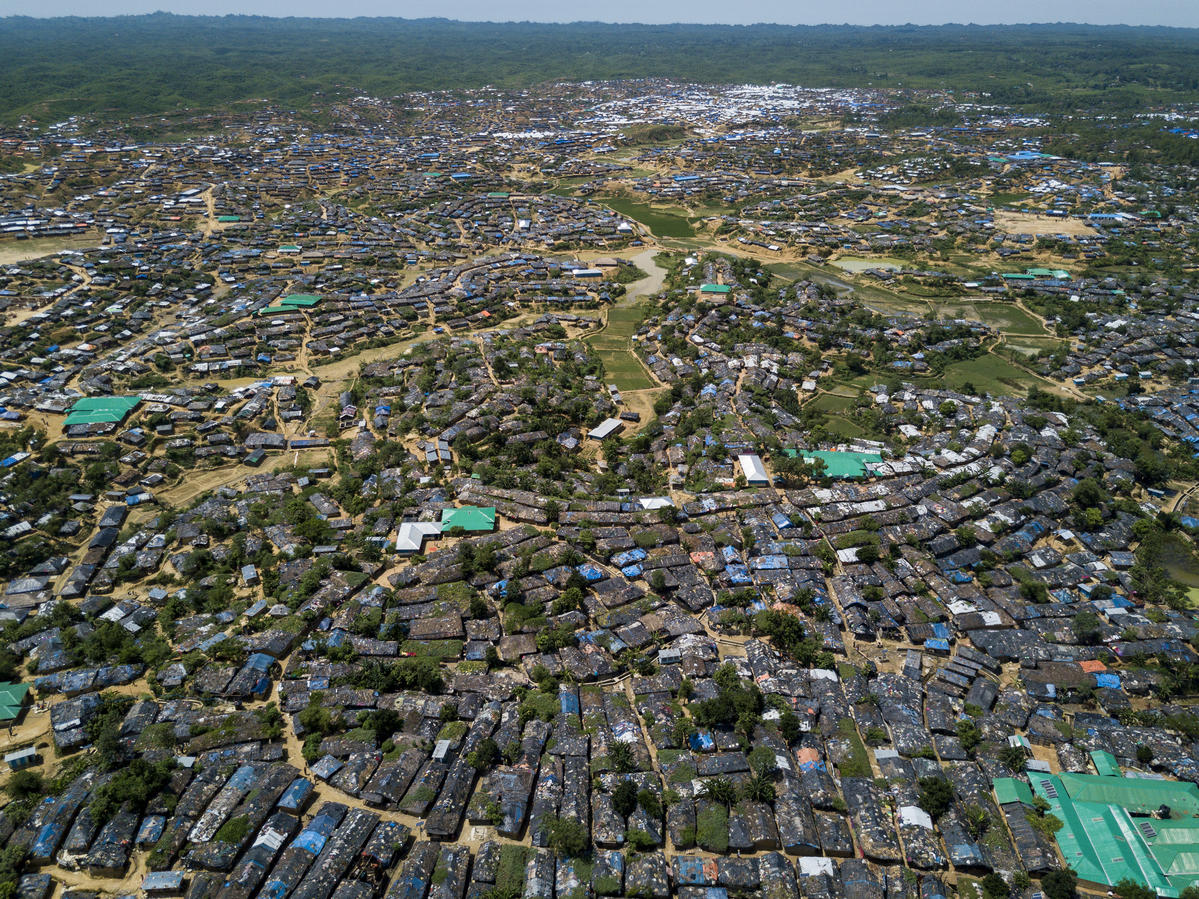
On 25 August 2017, a deadly Army crackdown in Myanmar’s Rakhine State forced thousands of stateless Rohingya to flee across the border to Bangladesh in search of refuge. Over the next 100 days, some 620,000 Rohingya fled the violence and widespread human rights violations, making it one of the fastest growing humanitarian crises. The sudden and massive flow of Rohingya into Bangladesh – as many as thousands a day – stretched UNHCR and humanitarian organizations to the limits of their capacities.
The event is just one of many situations during which having reliable data would have assisted UNHCR and UN agencies in preparing high-quality projections and contingency planning for emergencies. But such data are often either unavailable or only available with a considerable time lag, which renders them useless for operations and emergency preparedness.
The potential of ‘big data’ lies primarily in the application of insights from new data sources to inform policy interventions. The data revolution has been driven by explosions in the volume of data, the speed with which data are produced, the number of producers of data, and the range of issues on which there is data. This data is generated by new technologies such as mobile phones and social media and can be integrated with traditional data to produce high-quality information that is detailed, timely, and relevant. These shifts also create opportunities to improve data openness and transparency, which must be leveraged in a way that protects the individuals’ rights to privacy and does no harm.
The potential of big data to facilitate early warning of crises
lies in its ability to provide granular, almost real-time information
in locations where there are few other data sources.
The potential of big data to facilitate early warning of emerging issues and crises lies in its intrinsic ability to provide more granular, near real-time information, especially in locations where other sources of data are lacking. Some big data sources, such as satellite imagery, data from search queries (e.g. Google Trends), or data from traditional media or social media, can have the power to fill some of the information gaps left by conventional data acquisition channels, especially during crisis situations. Sentiment analyses from social media data, or ‘buzz‘, the intensity with which a topic is discussed in the media, or large-scale population movements on the ground captured by high resolution satellite images, all have the potential to improve existing early warning systems.
Indeed, several case studies have demonstrated that the inclusion of such data sources significantly improves the power and accuracy of predictive models of refugee and internal displacement flows (e.g., Agrawal, et al., 2016). A study on internally displaced persons (IDPs) movements between provinces in Iraq by Singh, et al. (2019) shows that a mix of social media data and traditional register data improves the predictive quality in comparison to predictions based on register data alone. Maybe even more impressive, though, is the predictive power of social media data in comparison to that of traditional register data that do not include the essential variable ‘death counts’. As the authors show, ‘event buzz’, the intensity with which events are discussed on social media, are highly correlated with death counts. As ‘event buzz’ can be captured in near-real time, thereby greatly increasing the timeliness of predictions of population movements, particularly in crisis situations.
However, like all data sources, big data come with caveats. Issues with big data mostly come from three sources: bias, inaccuracy, and low scalability (the possibility to extend a particular data source globally or to a vast array of different situations).
Big data hold huge potential for humanitarian organisations
to improve their early warning systems and generate
better contingency planning figures.
But they are no magic bullet.
Many big data sources rely on digital data streams and raw user content. The latter requires access to digital devices like computers, smart mobile phones, and the internet. Although penetration rates of such devices are increasing globally, they still do not reach 100% of the world population. Remote rural communities, women, children, the illiterate, and the elderly are population segments that are underrepresented and risk of becoming invisible in big data sources.
In addition to a potential bias in the data, raw user content often generates challenges regarding the accuracy of the information content. Social media data is especially prone to fake news, inaccurate information, rumours, spam, and trolling. Locating social media posts geographically poses a further challenge, and studies show that it is often unclear whether user content on social media actually reflects the views and expressions of refugees, IDPs or of other groups who are of no interest to humanitarian organisations.
Finally, extending big data sources to a global early warning system or to a vast array of different situations can add to the challenge as the practical scalability of some big data sources is limited. Call Detail Records for example, which register users’ mobile phone usage and can be used to generate highly accurate maps of population movements, are proprietary to the carrier network and cannot be accessed unless a bilateral agreement between the analyst and the carrier network is in place. If the population movements of interest cross several borders, the number of agreements needed rises and quickly reaches its limit.
To conclude, big data hold huge potential for humanitarian organizations to improve their early warning systems and to generate better contingency and population planning figures. But they are no magic bullet. As with traditional data sources, analysts are well served to keep the shortcomings of every big data source in mind and to carefully evaluate the relevance, accuracy, and contribution of different big data sources for a given problem at hand.
For a more comprehensive review of big data sources, and of the opportunities to employ them to better predict forced displacement situations, please consult the paper recently published by the Global Data Service.